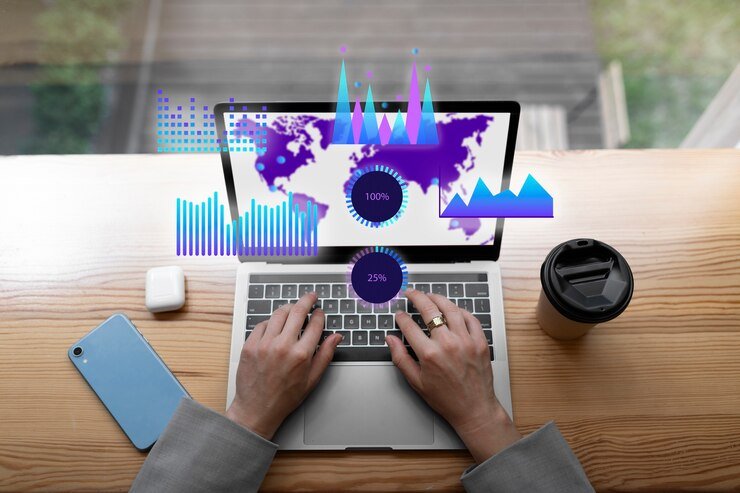
Introduction to data analysis
Data is everywhere, and understanding it can unlock valuable insights. When you encounter a set of numbers ranging from 17.4-9.8, what do you do? Whether it’s sales figures, survey results, or scientific measurements, analyzing this data effectively can make all the difference in decision-making.
In today’s digital landscape, data analysis isn’t just a skill; it’s essential for success across industries. This blog post will guide you through the process of dissecting and interpreting your numerical treasures. We’ll explore how to turn raw numbers into meaningful information that drives action and strategy.
Ready to dive into the world of data analysis? Let’s get started!
Understanding the Data Range
Data range is a fundamental concept in data analysis. It refers to the spread between the highest and lowest values in a dataset. For instance, when analyzing numbers like 17.4 and 9.8, understanding their relationship can unveil significant insights.
This range not only indicates variability but also highlights how concentrated or dispersed your data points are. A narrow range suggests consistency, while a broader one may signal diversity among observations.
Determining the data range helps set the stage for further statistical calculations. It guides analysts as they delve deeper into trends and patterns within the dataset. Whether you’re assessing performance metrics or sales figures, grasping these extremes can significantly enhance your strategic decisions.
By examining this simple yet powerful measure, you create a solid foundation for comprehensive analysis that drives results.
The Importance of Analyzing Data
Analyzing data is crucial in today’s information-driven world. It transforms raw numbers into valuable insights. These insights can drive decision-making across various sectors.
Businesses rely on data analysis to understand customer behavior and preferences. This understanding helps tailor products and services effectively.
In the field of healthcare, analyzing patient data leads to improved treatment outcomes. Patterns identified through analysis can inform better practices and innovations.
Education also benefits from careful examination of performance metrics. By assessing student achievement, educators can adapt techniques for enhanced learning experiences.
Moreover, effective data analysis fosters transparency and accountability in organizations. It allows stakeholders to track progress against objectives, ensuring everyone stays aligned with goals.
Recognizing trends within datasets empowers individuals and institutions alike to make informed choices that propel success forward.
Step-by-Step Guide to Analyzing Data Ranging from 17.4 to 9.8
Analyzing data can feel overwhelming, but breaking it down into manageable steps simplifies the process.
Start by organizing your data effectively. Group similar values together and create a clear layout to make analysis easier.
Next, calculate key statistics like the mean, median, and mode. These figures provide insight into trends within your range of 17.4 to 9.8.
After that, assess the range and standard deviation to understand variability in your dataset. This will help you gauge how spread out or clustered your data points are.
Bring your findings to life with visual representations such as graphs and charts. Visual aids not only enhance understanding but also reveal patterns that numbers alone might obscure.
Engaging with these methods will deepen your comprehension of the data at hand while making it accessible for others too.
A: Organize the Data
Organizing your data is the foundation of effective analysis. Before diving into calculations, take a moment to sort everything out.
Start by collecting all relevant data points ranging from 17.4 to 9.8. You might have numbers scattered across spreadsheets or notes—gather them in one place for clarity.
Next, decide on an appropriate format for your dataset. Consider using tables or lists that allow easy comparisons and insights later on.
Label each column clearly, making it simple to identify categories like time periods, specific observations, or even demographic information if applicable.
Once everything is arranged neatly, review for any inconsistencies or duplicates. Clean datasets lead to accurate conclusions and help avoid confusion down the line.
With organized data at hand, you’re ready to move forward with deeper analyses and discover meaningful patterns within those numbers.
B: Calculate the Mean, Median, and Mode
Calculating the mean, median, and mode can illuminate your data’s story. These three metrics offer different insights that complement each other.
First, the mean is straightforward. Add up all your numbers between 17.4 and 9.8, then divide by how many values you have. This gives you a sense of average performance or measurement.
Next up is the median. To find it, organize your numbers from smallest to largest. The center value represents where half of your data points lie above and below this point—a vital indicator of central tendency that’s resistant to outliers.
Don’t overlook the mode—the most frequently occurring number in your dataset. In some instances, especially with repeated measures or categories within 17.4-9.8 range calculations, this metric helps identify trends not captured by mean or median alone.
These calculations provide a clearer picture of underlying patterns within your data set.
C: Determine the Range and Standard Deviation
Determining the range is a straightforward yet crucial step in data analysis. To find it, simply subtract the smallest value from the largest one. In this case, you would take 17.4 and subtract 9.8, yielding a range of 7.6. This number gives you insight into how spread out your data points are.
Next up is standard deviation, which measures how much variation exists from the average (mean). A low standard deviation indicates that most values cluster around the mean, while a high standard deviation suggests more dispersion.
To calculate it, follow these steps: first find the mean of your dataset; then determine each value’s distance from that mean and square those distances; finally, calculate their average before taking the square root of that result. These calculations will provide deeper insights into your dataset’s behavior between 17.4 and 9.8.
D: Create Visual Representations with Graphs and Charts
Visual representations breathe life into your data. They transform numbers into insights that are easily digestible.
Graphs and charts can simplify complex information. A well-constructed bar graph allows you to compare values at a glance, while line graphs showcase trends over time. Pie charts effectively illustrate proportions within your dataset.
Tools like Excel or Google Sheets make it easy to create these visuals. Just plug in your calculations from the previous steps and let the software do the heavy lifting.
Remember, clarity is key. Choose colors wisely to enhance readability without overwhelming viewers. Each visual should tell a story that highlights critical patterns or anomalies in your data ranging from 17.4 to 9.8.
With effective graphics, you’re not just presenting numbers; you’re conveying meaning, making it easier for stakeholders to understand and act on the findings.
Methods for analyzing data ranging from 17.4 to 9.8
When analyzing data that ranges from 17.4 to 9.8, several methods can provide valuable insights.
Start with basic calculations like the mean, median, and mode. The mean offers an average value, while the median gives you a midpoint of your dataset. Identifying the mode reveals which number appears most frequently.
Next, consider calculating standard deviation. This metric helps gauge how much individual data points deviate from the mean—a crucial aspect for understanding variability in your dataset.
Visual representations are equally important. Histograms allow you to see frequency distribution clearly, while box plots offer a concise view of data spread and potential outliers.
Using these methods enables deeper insight into your dataset’s characteristics and trends without overwhelming complexity or ambiguity in interpretation.
– Mean, median, and mode calculations
When analyzing data points, calculating the mean, median, and mode is essential. These three measures provide a comprehensive understanding of your dataset.
The mean is simply the average. You add all values together and divide by the number of observations. It gives you a quick snapshot but can be influenced by outliers.
Next comes the median. This value represents the middle point when data is arranged in ascending order. It’s particularly useful when your dataset includes extremes that skew results.
We have mode—the most frequently occurring value in your set. In some cases, there might be more than one mode or none at all if no number repeats.
Each measure tells its own story about your data’s distribution and characteristics. Understanding these calculations deepens insight into what lies behind those numbers.
– Standard deviation
Standard deviation is a key statistical measure that reflects the dispersion of data points in a dataset. It tells us how spread out the values are around the mean. A smaller standard deviation indicates that the data points tend to be close to the mean, while a larger one signifies more variability.
Calculating standard deviation involves several steps. First, you find the mean of your dataset. Then, each data point’s difference from this average is squared and summed up. You divide by the total number of observations and take the square root.
This metric becomes especially useful when analyzing datasets ranging from 17.4 to 9.8 because it helps identify consistency or volatility within those values. Understanding how much variability exists can inform decision-making processes across various fields such as finance, research, and quality control in manufacturing settings.
– Histograms and box plots
Histograms and box plots are powerful tools for data visualization. Each serves a unique purpose in analyzing your dataset.
A histogram displays the frequency distribution of numerical data. It uses bars to represent how many values fall within specified ranges, known as bins. This method allows you to quickly identify patterns, revealing whether your data is skewed or normally distributed.
Box plots offer another perspective by summarizing key statistics of your dataset. They depict the median, quartiles, and potential outliers in a compact format. By comparing multiple box plots side-by-side, you can easily assess differences between datasets or groups.
Both visuals enhance understanding when working with numbers ranging from 17.4 to 9.8. They provide clarity that raw figures often lack, making it easier to derive insights from complex information while facilitating effective decision-making processes.
Interpreting the results and identifying patterns or trends
Interpreting results is a crucial step in data analysis. Once you’ve calculated means, medians, and standard deviations, the next challenge lies in making sense of these numbers.
Look for patterns that emerge from the data ranging from 17.4 to 9.8. Are there any noticeable clusters or gaps? Patterns can reveal insights into behavior or trends over time.
Consider segmenting your findings based on different variables. This may help identify correlations or anomalies within specific groups.
Visual aids such as graphs and charts can also enhance interpretation. They make it easier to spot trends at a glance and provide clarity that raw data often lacks.
Always remain open-minded about what the data reveals. Unexpected results might lead to new hypotheses worth exploring further, opening doors to deeper understanding in your analysis journey.
Real-world examples and applications of analyzing this type of data
Analyzing data ranging from 17.4 to 9.8 has practical implications across various fields. In healthcare, researchers often examine patient recovery scores that fall within this range to identify effective treatment protocols.
In education, teachers might analyze student test scores to pinpoint strengths and weaknesses in curriculum design. A score of 17.4 could indicate exceptional performance while a 9.8 may highlight areas needing improvement.
Marketing teams utilize this data analysis for customer feedback ratings, helping them refine products or services based on user satisfaction levels.
Sports analysts look at player performance metrics within the same scale, assessing how individual contributions impact team success over time.
Environmental scientists study pollution levels that fluctuate between these numbers to understand trends and devise strategies for mitigation efforts effectively.
Conclusion: The value and impact of
Analyzing data ranging from 17.4 to 9.8 offers valuable insights across various fields. By understanding the intricacies of this data, you can uncover patterns that inform decisions and strategies.
The process of organizing your data sets the foundation for meaningful analysis. Calculating mean, median, mode, range, and standard deviation provides a clearer picture of your dataset’s characteristics. Visual representations like histograms and box plots enhance comprehension by making complex information more accessible.
Real-world applications are abundant: businesses can optimize operations based on customer feedback scores within this range; educators may assess student performance metrics to improve teaching methods; researchers often analyze experimental results for validity.
The ability to distill meaning from numerical values like those between 17.4 and 9.8 transforms raw numbers into actionable intelligence that drives progress in various sectors.
RELATED POSTS
View all